The Emergence of AIoT: Threats and Solutions
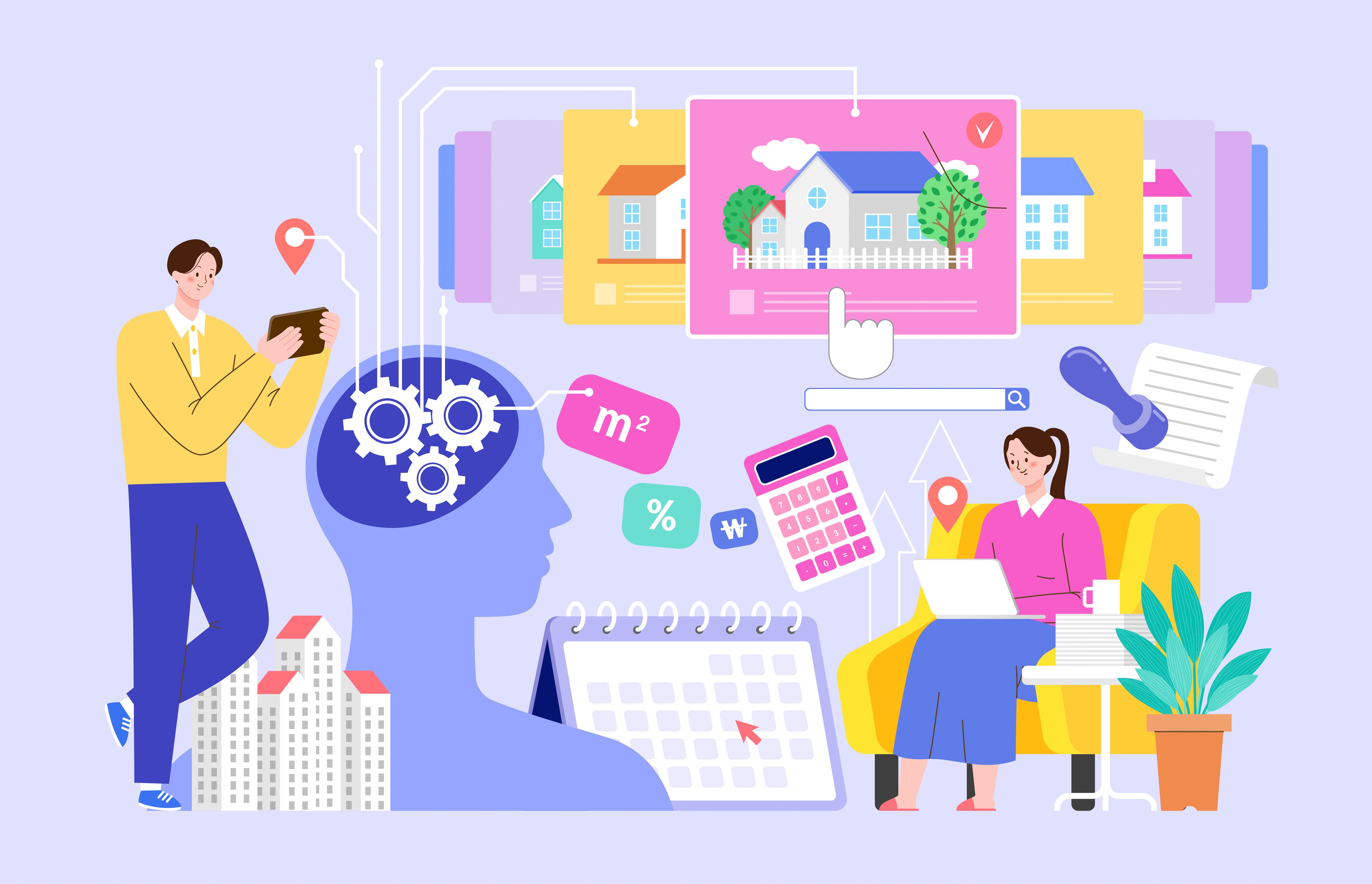
The expansive reach of IoT (Internet of Things) technology into diverse realms such as smart glasses, home appliances, and smart farming is revolutionizing our lives, making them more intelligent and convenient. According to Transforma Insights, as of 2023, approximately 15 billion digital devices globally are connected via IoT, a figure projected to surge to about 30 billion by 2030.
Source: Transforma Insights
In the era of generative AI, a pivotal development to observe is the convergence of artificial intelligence (AI) advancements with traditional IoT devices like home appliances. Devices are evolving from simply being interconnected and performing tasks based on predefined algorithms to making autonomous decisions. This evolution marks the rapid transition to AIoT (AI Internet of Things).
However, AIoT, the epitome of cutting-edge technology, exposes serious security vulnerabilities. This stems from its dual nature: the inherent characteristics of IoT that connect numerous devices via networks, and its reliance on vast data sets and machine/deep learning algorithms. This article aims to delve into the core concepts of AIoT, identify the root causes of these issues, and introduce strategies for preparing robust security measures in the age of AIoT.
1. What is AIoT, a Fusion of AI and IoT?
AIoT (Artificial Intelligence of Things) refers to an integrated technology where data collected through the Internet of Things (IoT) is analyzed by artificial intelligence algorithms, enabling autonomous decision-making. AIoT, also known as "object intelligence" or "composite object intelligence," holds immense potential to enhance our lives by making intelligent decisions based on the data collected by various objects.
So, what distinguishes AIoT from traditional IoT? The key difference lies in their operational approach. While IoT operates based on pre-defined programs and algorithms, AIoT performs intelligent operations through learning and inference.
In devices equipped with traditional IoT technology, the role is limited to collecting data through sensors and executing specified commands. In contrast, a smart home utilizing AIoT applies machine learning-based AI algorithms to the collected data, learning from user behaviors and preferences, and sensing the environment to provide optimal convenience and energy efficiency.
For instance, consider a scenario where a user sends commands to turn lights on and off via a smartphone app in a traditional IoT system. The lighting sensor in the IoT setup detects the intensity of light and transmits this information to a central server. The central server then controls the lighting based on set rules and responds to the user. Such conventional IoT systems operate on fixed rules and have limited capacity to adapt to changes in user habits or environmental conditions.
On the other hand, a smart home system with AIoT is far more intelligent. When a user sends commands to control lighting via a smartphone app, the lighting sensor can collect not only the intensity of light but also the user's behavior patterns and preferences. Moreover, the built-in machine learning algorithms analyze this data to learn the user's habits, enabling the prediction of future behavior and thus offering an optimized living environment.
Furthermore, lighting control in AIoT systems can occur both through commands from a central server and internally within the device, dynamically adjusting based on learned patterns. For example, if the AI algorithm learns that the user frequently turns on the lights at a certain time, it may autonomously decide to turn on the lights automatically at that time.
2. Security Issues Arising from the Proliferation of AIoT
The convergence of artificial intelligence and IoT undeniably offers unprecedented experiences and conveniences. However, it also introduces a new level of security concerns, surpassing those associated with traditional IoT.
-Data Privacy and the Black Box Model Issue
IoT technology inherently risks exposing sensitive information due to its capacity to collect and analyze vast amounts of personal data through sensors, potentially leading to privacy breaches. This issue is particularly pronounced in AIoT, where, in addition to the security technologies of IoT, decision-making is entrusted to artificial intelligence algorithms, raising concerns about AI ethics and the opacity of these models, often referred to as the "black box" problem.
This issue is closely related to the operational mode of AIoT. Unlike traditional IoT, AIoT relies on machine learning algorithms to infer user behavior. When deep learning algorithms, especially those based on Deep Neural Networks (DNN), are used to detect patterns within large datasets, AIoT systems learn and make decisions based on user data. The complexity of these decisions, often lacking transparency for the users, leads to the black box problem, where the reasoning behind these decisions is not readily understandable or resolvable by the users.
- Cyber Attacks on Devices and Systems
Like traditional IoT, AIoT can become a target for cyber attackers. This vulnerability often arises from the lack of a robust authentication system among connected devices, allowing attackers to integrate fake devices into the system, thereby disrupting its normal operation.
For example, in AIoT, a Distributed Denial-of-Service (DDoS) attack, which prevents the system from utilizing its resources as planned, can not only cause inconvenience due to sudden device malfunctions but also pose a risk of safety incidents. Given the nature of IoT where devices are interconnected and exchange data through networks, the scale of damage can be significant when these devices become targets of malicious cyber attacks through viruses or malware. Therefore, heightened vigilance is essential to mitigate these risks.
- Data Tampering and Manipulation
Hackers can also create chaos within artificial intelligence systems by tampering with or manipulating the collected data. Considering the nature of supervised learning-based AI algorithms, which make decisions based on learned data, the risk of data manipulation is significant. Such tampering can not only cause inconvenience to users by leading to erroneous decisions by the AI model but also potentially expose them to danger.
3. Solutions to Security Issues Related to AIoT:
- Strengthening Data and Device Security
Ensuring data security is critical for user privacy and safety. When sensitive personal information is collected, it is essential to obtain user consent, clearly define and adhere to data collection and utilization policies, and implement strong data encryption technologies to guarantee data security.
Similarly, for user safety, adopting robust authentication and encryption technologies for secure communication between devices, and providing regular security updates and patches to minimize vulnerabilities, are vital steps.
Implementing SSenStone's OTAC for Device Authentication Systems
One of solutions to enhance data and device security is OTAC (One-Time Authentication Code). SSenStone has developed OTAC, an groundbreaking authentication method surpassing the limitations of traditional methods, and establishing a new standard in authentication security.
SSenStone's OTAC is the world’s first unidirectional dynamic authentication technology, supporting real-time, always-changing, one-time authentication even in off-the-network environments for both user and device identification. Importantly, each generated code is unique to each user, ensuring a more secure authentication environment.
OTAC technology combines the advantages of the three most commonly used authentication systems: username and password, RSA hardware and software for authentication code generation, and tokenization. This integration offers a more efficient and effective authentication process, capable of generating unidirectional dynamic codes for identification and authentication of users and devices, even in environments with no or unstable communication networks.
The generated codes are one-time use and designated for legitimate users within specific time frames, making them unusable or non-reusable by others. With OTAC, a robust device authentication system can be established to control unauthorized devices, enhancing identity verification of devices to block illegal access and maintain system security.